SESSION + Live Q&A
Tools to Put Deep Learning Models in Production
While there are a lot of machine learning frameworks and libraries available, putting the models in production at large scale is still a challenge. I’d like to talk about how we took on the challenge of supporting the data scientists with their efforts by making it easy to put their models in production. I’ll be covering how we:
- chose our tools and developed the internal deep learning infrastructure
- train our models in docker containers
- automate the re-training of models
- deploy models using Kubernetes
I’ll also talk about how we optimize our model prediction infrastructure for latency or throughput depending on the use case.
Speaker

Sahil Dua
Developer at Booking.com; Open Source Contributor in DuckDuckGo, GitHub and Pandas
Sahil is a software developer at Booking.com. He has been involved in leveraging container infrastructure to help Booking.com’s internal teams in taking advantage of deep learning techniques at scale. An open source software enthusiast, Sahil is a core contributor and community leader for...
Read moreFind Sahil Dua at:
From the same track
Fuelling the AI Revolution with Gaming
Artificial Intelligence will improve productivity, products and services, across a broad range of applications, all benefiting humanity. NVIDIA is researching all areas and working closely with top research labs around the world, Enterprise & startups in both problem-solving and getting...
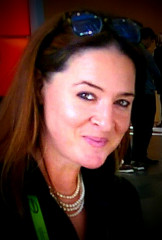
Alison Lowndes
Artificial Intelligence DevRel @NVIDIA
Models in Minutes not Months: AI as Microservices
Companies are redefining their businesses by building models and learning from data. Whether it is using data science to predict their best sales and marketing targets, automating digital customer interactions using bots, or reducing waste in logistics and manufacturing - Artificial Intelligence...
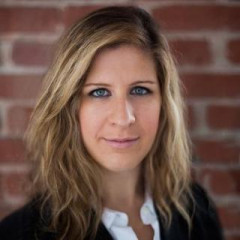
Sarah Aerni
Director, Data Science @Salesforce Einstein
AI in the Asset Management Industry
In the Financial industry, Artificial Intelligence has been one of the sophisticated techniques used by early adopters to manage multiple assets. Those early adopters are Quantitative Hedge Funds, around since the 80s and managing today an estimated USD 940 billion. After presenting the main...
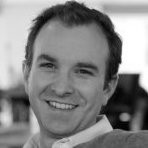
Antoine Pichot
Quantitative Researcher @Systematica Investments
Machine Intelligence at Google Scale
The biggest challenge of Deep Learning technology is the scalability. As long as using single GPU server, you have to wait for hours or days to get the result of your work. This doesn't scale for production service, so you need distributed training on the cloud eventually, or take advantage of...
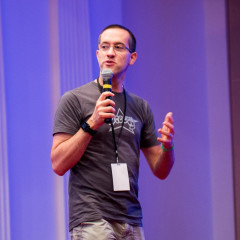
Guillaume Laforge
Developer Advocate at Google Cloud and PMC Chair for Apache Groovy
AI Panel
Join the track speakers and invited guests as they discuss where AI is heading and how it's affecting software today.

Sahil Dua
Developer at Booking.com; Open Source Contributor in DuckDuckGo, GitHub and Pandas
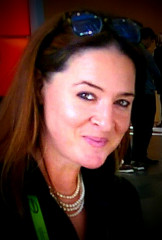
Alison Lowndes
Artificial Intelligence DevRel @NVIDIA
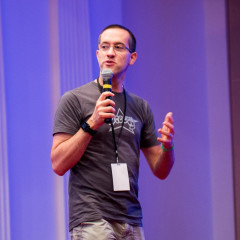
Guillaume Laforge
Developer Advocate at Google Cloud and PMC Chair for Apache Groovy
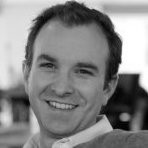
Antoine Pichot
Quantitative Researcher @Systematica Investments
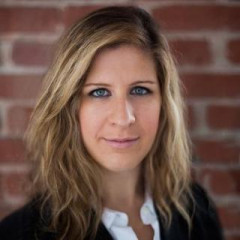
Sarah Aerni
Director, Data Science @Salesforce Einstein
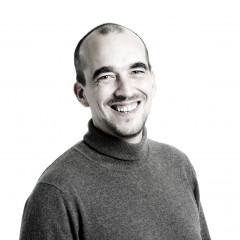
Eric Horesnyi
CEO @streamdata.io
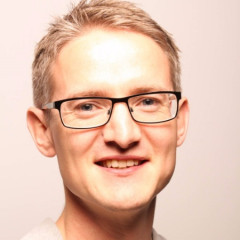
Philip Winder
Consultant, Engineer, Scientist @Winder Research and Development Ltd.