SESSION + Live Q&A
Building Data Pipelines in Python
This talk discusses the process of building data pipelines, e.g. extraction, cleaning, integration, pre-processing of data, in general all the steps that are necessary to prepare your data for your data-driven product. In particular, the focus is on data plumbing and on the practice of going from prototype to production.
Starting from some common anti-patterns, we'll highlight the need for a workflow manager for any non-trivial project.
We'll discuss the case for Luigi as an interesting option to consider, and we'll consider where it fits in the bigger picture of deploying a data product.
Speaker
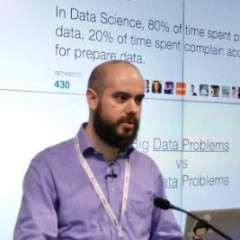
Marco Bonzanini
Data Scientist & Co-Organiser of PyData London Meetup
I'm a Data Science consultant based in London, UK. Author of "Mastering Social Media Mining with Python", published by Packt Publishing. Co-organiser of the PyData London meetup. Backed by a PhD in Information Retrieval, I specialise in search applications and text analytics applications, and...
Read moreFind Marco Bonzanini at:
From the same track
Effective Data Pipelines: Data Mngmt from Chaos
Creating automated, efficient and accurate data pipelines out of the (often) noisy, disparate and busy data flows used by today's enterprises is a difficult task. Data science teams and engineering teams may be asked to work together to create a management platform (or install one) that helps...
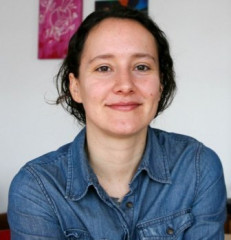
Katharine Jarmul
Python engineer, Founder @kjamistan
Data Cleansing and Understanding Best Practices
Any data scientist who works with real data will tell you that the hardest part of any data science task is the data preparation. Everything from cleaning dirty data to understanding where your data is missing and how your data is shaped, the care and feeding of your data is a prime task for the...
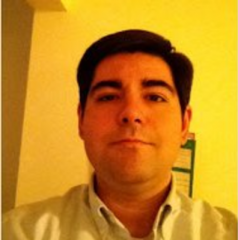
Casey Stella
Committer and PMC member on the Apache Metron project
Reliable & Scalable Data Infra Eco-System At Uber
Uber's vision is to make transportation as reliable as running water everywhere, for everyone. Data is key for Uber's 24x7 global business operations and making data available for different use cases across the company in a reliable, scalable and performant way is often challenging. In this...
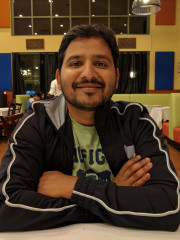
Sudhir Mallem
Staff Engineer @Uber
Building a Data Science Capability From Scratch
This talk will cover the challenges, both technical and cultural, of building a data science team and capability in a large, global company. It will discuss best practices, lessons learned, and rewards of leveraging data effectively in the next frontier of data science: commercial insurance.
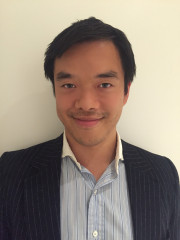
Victor Hu
Head of Data Science @QBE