SESSION + Live Q&A
Reliable & Scalable Data Infra Eco-System At Uber
Uber's vision is to make transportation as reliable as running water everywhere, for everyone. Data is key for Uber's 24x7 global business operations and making data available for different use cases across the company in a reliable, scalable and performant way is often challenging.
In this talk, we will discuss the overall data analytics eco-system at Uber and learn on how Uber shapes its data from a raw form to a modeled form by leveraging various in-house and open source technologies such as Hadoop, Hive on Tez/MR, Spark, Presto, Airflow and Enterprise technology such as HPE Vertica. Consumers of this data include Machine Learning & data science, city operations, Experimentation, Fraud, Marketplace and Growth Analytics.
We will also discuss on a whole different aspect of going back to basics on traditional data modeling and how it has helped us scale analytical and adhoc interactive queries while retaining the same standard SQL interface offered by SQL-on-Hadoop technologies like Hive, Presto and Spark. We will also discuss how we built and orchestrate ETL and Data processing pipelines leveraging Piper (forked from Airflow).
Finally, we will discuss couple of real time use cases of leveraging this framework and how this helped us power key business operations.
Speaker
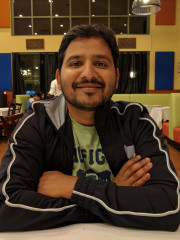
Sudhir Mallem
Staff Engineer @Uber
Sudhir Mallem is a Staff Engineer at Uber working in the data infrastructure team. He was previously a Staff engineer and an early team member of the data infra team at LinkedIn where he built and maintained massively scalable enterprise and analytical warehouse that powered business operations,...
Read moreFind Sudhir Mallem at:
From the same track
Effective Data Pipelines: Data Mngmt from Chaos
Creating automated, efficient and accurate data pipelines out of the (often) noisy, disparate and busy data flows used by today's enterprises is a difficult task. Data science teams and engineering teams may be asked to work together to create a management platform (or install one) that helps...
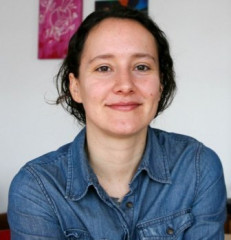
Katharine Jarmul
Python engineer, Founder @kjamistan
Data Cleansing and Understanding Best Practices
Any data scientist who works with real data will tell you that the hardest part of any data science task is the data preparation. Everything from cleaning dirty data to understanding where your data is missing and how your data is shaped, the care and feeding of your data is a prime task for the...
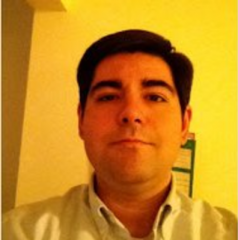
Casey Stella
Committer and PMC member on the Apache Metron project
Building a Data Science Capability From Scratch
This talk will cover the challenges, both technical and cultural, of building a data science team and capability in a large, global company. It will discuss best practices, lessons learned, and rewards of leveraging data effectively in the next frontier of data science: commercial insurance.
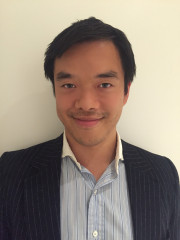
Victor Hu
Head of Data Science @QBE
Data Engineering Open Space
Building Data Pipelines in Python
This talk discusses the process of building data pipelines, e.g. extraction, cleaning, integration, pre-processing of data, in general all the steps that are necessary to prepare your data for your data-driven product. In particular, the focus is on data plumbing and on the practice of going from...
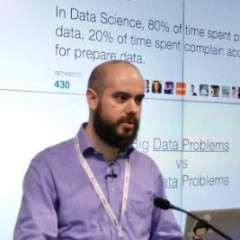
Marco Bonzanini
Data Scientist & Co-Organiser of PyData London Meetup