SESSION + Live Q&A
Test Driven Machine Learning
Software engineers are familiar with test driven development, but are not familiar with the statistical testing required in machine learning. Machine learning specialists are familiar with testing during the model building phase when they withhold data for cross-validation or final testing, but they are unfamiliar with software engineering principles. While testing a learned model gives an idea how well it might perform on unseen data it is not sufficient for model deployment. Trying to learn from test driven development practices we are looking across the machine learning life cycle to understand where we need to test and how this can be done. The testing of data, for example, is essential as it not only drives the machine learning phase itself, but it is paramount for producing reliable predictions after deployment. Testing the decisions made by a deployed machine learning model is equally important to understand if it delivers the expected business value.
Speaker
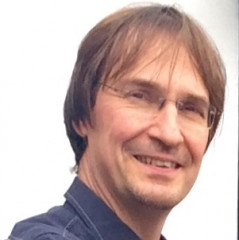
Detlef Nauck
Chief Research Scientist for Data Science @BTGroup and Visiting Professor @bournemouthuni
Detlef Nauck is Chief Research Scientist for Data Science with BT's Research and Innovation Division located at Adastral Park, Ipswich, UK. He is leading a group of international scientists working on research into Data Science, Machine Learning and AI. Detlef focuses on establishing best...
Read moreFind Detlef Nauck at:
From the same track
How to Prevent Catastrophic Failure in Production ML Systems
AI systems can fail catastrophically and without warning, a characteristic not welcomed in the corporate environment. Martin will describe the unpredictable nature of artificial intelligence systems and how mastering a handful of engineering principles can mitigate the risk of failure. You’ll...
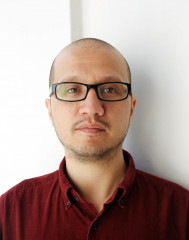
Martin Goodson
Chief Scientist/CEO @EvolutionAI
H2O's Driverless AI: An AI that creates AI
Through my kaggle journey to the top spot, I have noticed that many of the things I do as a data scientist can be automated. In fact automation is critical to achieve good scores and promote accountability, ensuring that common pitfalls in the modelling process are prevented. Through...
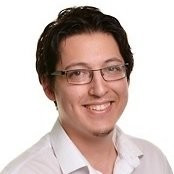
Marios Michailidis
Competitive Data Scientist @h2oai
Intuition & Use-Cases of Embeddings in NLP & Beyond
Machine Learning has achieved tremendous advancements in language tasks over the last few years (think of technologies like Google Duplex, Google Translate, Amazon Alexa). One of the fundamental concepts underpinning this progress is the concept of word embeddings (using something like the...
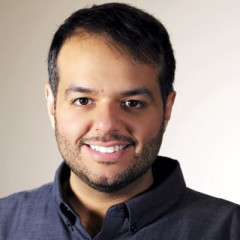
Jay Alammar
VC and Machine Learning Explainer @STVcapital
Understanding Deep Learning
No matter what your role is, it is really important to have some understanding of the models you’re working with. In last year's keynote, Rob Harrop talked about the importance of intuition in machine learning. This is a step towards that. You might already be using neural networks. How can...
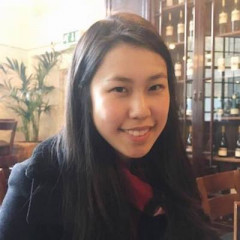
Jessica Yung
Machine Learning blogger and entrepreneur, Self-Driving Car Engineer Scholar @nvidia
AI/Machine Learning Open Space
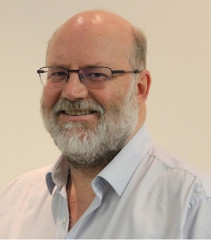
Shane Hastie
Director of Agile Learning Programs @ICAgile